引用本文: | 佴兆骏,段洪涛,朱利,曹志刚,陈晓宁,张玉超,马荣华.基于环境卫星CCD数据的太湖蓝藻水华监测算法研究.湖泊科学,2016,28(3):624-634. DOI:10.18307/2016.0319 |
| NAI Zhaojun,DUAN Hongtao,ZHU Li,CAO Zhigang,CHEN Xiaoning,ZHANG Yuchao,MA Ronghua.A novel algorithm to monitor cyanobacterial blooms in Lake Taihu from HJ-CCD imagery. J. Lake Sci.2016,28(3):624-634. DOI:10.18307/2016.0319 |
|
|
|
本文已被:浏览 7761次 下载 3093次 |
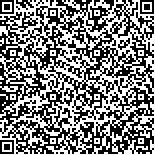 码上扫一扫! |
|
基于环境卫星CCD数据的太湖蓝藻水华监测算法研究 |
佴兆骏1,2, 段洪涛2, 朱利3, 曹志刚2, 陈晓宁1, 张玉超2, 马荣华2
|
1.西安科技大学测绘科学与技术学院, 西安 710054;2.中国科学院南京地理与湖泊研究所湖泊与环境国家重点实验室, 南京 210008;3.环境保护部卫星环境应用中心, 北京 100094
|
|
摘要: |
水体富营养化引起的蓝藻水华问题,是我国湖泊面临的主要环境问题,亟需加强现状监测和变化研究;我国自主研发的环境(HJ)卫星空间分辨率高,重访周期短,可用于长时间序列蓝藻水华的动态监测.本文利用HJ卫星CCD数据,通过自动控制散点回归的方法进行相对辐射校正,再将归一化植被指数和像元生长算法相结合,提出了一种可业务化运行的蓝藻水华高精度提取算法.该算法的优点为:(1)水华提取时具有统一的阈值,解决了以往一景影像一个阈值,无法大规模批处理的难题;(2)通过对像元进行线性分解,精度可达到亚像元级别.利用该算法对太湖2009-2014年蓝藻水华进行监测,发现2013-2014年太湖蓝藻水华较以往暴发面积偏小.研究表明,该算法对蓝藻水华识别能力强,自动化程度和水华提取精度高,可作为业务化算法运行. |
关键词: 环境卫星 蓝藻水华 自动控制散点回归 归一化植被指数 像元生长算法 太湖 |
DOI:10.18307/2016.0319 |
分类号: |
基金项目:国家高技术研究发展计划"863"项目(2014AA06A509)资助. |
|
A novel algorithm to monitor cyanobacterial blooms in Lake Taihu from HJ-CCD imagery |
NAI Zhaojun1,2, DUAN Hongtao2, ZHU Li3, CAO Zhigang2, CHEN Xiaoning1, ZHANG Yuchao2, MA Ronghua2
|
1.College of Surveying and Mapping Science and Technology, Xi'an University of Science and Technology, Xi'an 710054, P. R. China;2.State Key Laboratory of Lake Science and Environment, Nanjing Institute of Geography and Limnology, Chinese Academy of Sciences, Nanjing 210008, P. R. China;3.Environment Satellite Center, Ministry of Environmental Protection, Beijing 100094, P. R. China
|
Abstract: |
Cyanobacterial blooms are common occurrences in eutrophic lakes, and it's urgent to monitor their area and extent effectively by remote sensing. HJ-CCD satellite data has high spatial and temporal resolution and is suitable for detecting cyanobacterial blooms of long time. In this study, relative radiometric correction of HJ-CCD images was processed using the Automatic Scattergram-Controlled Regression method to eliminate the radiometric problems. Then, a high-precision extraction algorithm of cyanobacterial blooms was put forward by combination of Normalized Difference Vegetation Index and Algae Pixel-growing Algorithm. This method provides an uniform threshold for the different HJ-CCD images and can extract blooms information of sub-pixel. Using this algorithm to monitor cyanobacterial bloom in Lake Taihu from 2009 to 2014, we found that the area of cyanobacterial blooms decreased during 2013 and 2014. The result indicated that this algorithm could identify the cyanobacteria bloom effectively and automatically with high accuracy. |
Key words: HJ-CCD satellite data cyanobacteria bloom Automatic Scattergram-Controlled Regression Normalized Difference Vegetation Index Algae Pixel-growing Algorithm Lake Taihu |
|
|
|
|