引用本文: | 丁艺鼎,蒋名亮,徐力刚,范宏翔,吕海深.基于鲸鱼优化算法的长短期记忆模型水库洪水预报.湖泊科学,2024,36(1):320-332. DOI:10.18307/2024.0143 |
| Ding Yiding,Jiang Mingliang,Xu Ligang,Fan Hongxiang,Lv Haishen.Flood forecasting method for reservoirs based on WOA-LSTM. J. Lake Sci.2024,36(1):320-332. DOI:10.18307/2024.0143 |
|
|
|
本文已被:浏览 2565次 下载 2298次 |
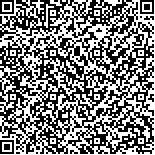 码上扫一扫! |
|
基于鲸鱼优化算法的长短期记忆模型水库洪水预报 |
丁艺鼎1,2,3, 蒋名亮2, 徐力刚2,4,3, 范宏翔2, 吕海深1
|
1.河海大学水文与水资源学院, 南京 210098;2.中国科学院南京地理与湖泊研究所, 南京 210018;3.江西省鄱阳湖流域生态水利技术创新中心, 南昌 330029;4.中国科学院大学南京学院, 南京 211135
|
|
摘要: |
洪涝灾害是世界主要自然灾害之一,优化洪水预报方案对防洪决策至关重要,然而传统水文模型存在参数多、调参受人为因素影响,泛化能力弱等问题。针对上述问题,本文提出基于改进的鲸鱼优化算法和长短期记忆网络构建自动优化参数的WOA-LSTM模型,通过优化神经网络结构进一步增强该模型的稳定性和精确度,并且建立不同预见期下的洪水预报模型来分析讨论神经网络结构与预报期之间的关系。以横锦水库流域1986—1997年洪水资料为例,其中以流域7个雨量站点的降雨以及横锦站水文资料为输入,不同预见期下洪水过程作为输出,以1986—1993年作为模型的率定期,1994—1997年作为模型的检验期,研究结果表明:(1)以峰现时差、确定性系数、径流深误差和洪峰流量误差作为评价指标,相比较于LSTM模型和新安江模型对检验期的模拟结果表明WOA-LSTM模型拥有更高的精度、预报结果更稳定;(2)结合置换特征值和SHAP法分析模型特征值重要性,增强了神经网络模型的可解释性;(3)通过改变神经网络结构在一定程度避免由于预见期增加和数据关联性下降而导致的模型预报精度下降的问题,最终实验表明该模型在预见期1~6 h下都可以满足横锦水库的洪水预报要求,可以为当地的防洪决策提供依据。 |
关键词: 洪水预报 长短期记忆模型(LSTM) 鲸鱼优化算法 深度学习 |
DOI:10.18307/2024.0143 |
分类号: |
基金项目:国家自然科学基金项目(41971137,U2240224,42001109)、江苏省自然科学基金项目(BK20201102)和江西省科技计划项目(20213AAG01012,20212BBG71002,20222BCD46002)联合资助。 |
|
Flood forecasting method for reservoirs based on WOA-LSTM |
Ding Yiding1,2,3, Jiang Mingliang2, Xu Ligang2,4,3, Fan Hongxiang2, Lv Haishen1
|
1.College of Hydrology and Water Resources, Hohai University, Nanjing 210098, P.R. China;2.Nanjing Institute of Geography & Limnology, Chinese Academy of Sciences, Nanjing 210018, P.R. China;3.Jiangxi Poyang Lake Basin Ecological and Water Conservancy Technology Innovation Center, Nanchang 330029, P.R. China;4.University of Chinese Academy of Sciences, Nanjing, Nanjing 211135, P.R. China
|
Abstract: |
Flood is one of the world's major natural disasters. Optimizing flood forecasting solutions is crucial for flood management decisions. However, traditional hydrological models suffer from issues such as a high number of parameters, susceptibility to human interference during parameter calibration, and weak generalization ability. To address these challenges, this study proposes a WOA-LSTM model that integrates an improved whale optimization algorithm and long short-term memory network to automatically optimize model parameters. Furthermore, the stability and accuracy of the model are further enhanced through the optimization of the neural network structure. Additionally, flood forecasting models with different lead times are established to analyze and discuss the relationship between the neural network structure and the forecast period. By selecting the Hengjin Reservoir basin as a case study, the rainfall data of seven stations and the hydrological data of Hengjin Station during 1986-1997 were collected as model inputs. The flood process under different forecast periods was model output with the calibration and validation periods of 1986-1993 and 1994-1997, respectively. The modeling results showed that: (1) Compared with the LSTM model and the XAJ model, the WOA-LSTM model achieved a better performance evaluated by the peak-present time difference, deterministic coefficient, relative error in runoff depth and peak flow error. (2) By displacing the eigenvalues, the SHAP method analyzed the importance of the model eigenvalues, and thus enhanced the interpretability of the neural network model. (3) By appropriately designing the neural network structure, the problem of model prediction accuracy deteriorating due to the decrease of data correlation caused by the increase of the forecast period can be avoided to a certain extent. The case study demonstrated that the model can meet the requirements of flood forecasting for Hengjin Reservoir during the forecast period of 1-6 h, and can support managers to achieve best management practices in controlling flood. |
Key words: Flood forecasting long-short term memory (LSTM) whale optimization algorithms deep learning |
|
|
|
|